报告主题:Challenges in applying Evolutionary Algorithms to real-world problems
报告人:LiXiaodong教授
报告时间:11月11日10:00
报告地点:航海学院201会议室
邀请人:彭星光教授
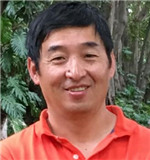
报告人简介:Xiaodong Li received his B.Sc. degree from Xidian University, Xi'an, China, and Ph.D. degree in information science from University of Otago, Dunedin, New Zealand, respectively. He is a full professor at the School of Science (Computer Science and Software Engineering), RMIT University, Melbourne, Australia. His research interests include evolutionary computation, neural networks, machine learning, complex systems, multiobjective optimization, multimodal optimization (niching), and swarm intelligence. He serves as an Associate Editor of the IEEE Transactions on Evolutionary Computation, Swarm Intelligence (Springer), and International Journal of Swarm Intelligence Research. He is a founding member of IEEE CIS Task Force on Swarm Intelligence, a Vice-chair of IEEE CIS Task Force of Multi-Modal Optimization, and a former Chair of IEEE CIS Task Force on Large Scale Global Optimization. He was the General Chair of SEAL'08, a Program Co-Chair AI'09, a Program Co-Chair for IEEE CEC’2012, a General Chair for ACALCI’2017 and AI’17. He is the recipient of 2013 ACM SIGEVO Impact Award and 2017 IEEE CIS “IEEE Transactions on Evolutionary Computation Outstanding Paper Award”.
报告内容简介:Evolutionary algorithms (or broadly speaking, meta-heuristics) represent a family of nature-inspired optimization techniques that have attracted much attention in recent years, due to their general applicability, robustness, and versatility. Many practitioners now realize EAs can offer significant advantages over exact methods, which often have strong assumptions. The adoption of EAs for solving difficult optimization problems is on the increase. However, there are still huge gaps when it comes to applying EAs to optimization problems considering more real-world characteristics. In the big data era, many practical problems are typically high dimensional, highly constrained, and combinatorial in nature. Furthermore, many optimization problems require the involvement of decision makers in the optimization process, rather than leave them out (i.e., human-in-the-loop). In this talk, I will describe some of my own experience in the past few years in dealing with applying evolutionary algorithms to several practical problems of this nature, including minimum cost network flow problems, resource scheduling mining problems, preference modelling for multi-criteria decision making. Since our primary goal is to design effective optimization techniques to solve problems of practical relevance, we deliberately avoided using test functions, typically constructed with too simplistic features far away from real-world scenarios. In this talk, I will focus on presenting the challenges we have encountered when tackling the above optimization problems